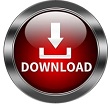
# Pretrained convolutional layers are loaded using the Imagenet weights. If set to 0, all pretrained layers will freeze during training Defaults to 'RMSProp'įine_tune: int - The number of pre-trained layers to unfreeze. Optimizer: string - instantiated optimizer to use for training. N_classes: int - number of classes for the output layer Input_shape: tuple - the shape of input images (width, height, channels) Once the pre-trained layers have been imported, excluding the "top" of the model, we can take 1 of 2 Transfer Learning approaches.ĭef create_model(input_shape, n_classes, optimizer='rmsprop', fine_tune=0):Ĭompiles a model integrated with VGG16 pretrained layers So we'll import a pre-trained model like VGG16, but "cut off" the Fully-Connected layer - also called the "top" model. The Fully-Connected layer generates 1,000 different output labels, whereas our Target Dataset has only two classes for prediction. Now we can't use the entirety of the pre-trained model's architecture. We can import a model that has been pre-trained on the ImageNet dataset and use its pre-trained layers for feature extraction. We know that the ImageNet dataset contains images of different vehicles (sports cars, pick-up trucks, minivans, etc.). Here's where Transfer Learning comes to the rescue! Writing our own CNN is not an option since we do not have a dataset sufficient in size. We want to generate a model that can classify an image as one of the two classes.
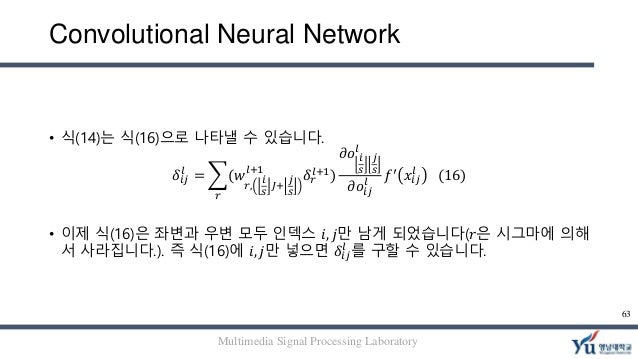
Now suppose we have many images of two kinds of cars: Ferrari sports cars and Audi passenger cars. The class probabilities are computed and are outputted in a 3D array (the Output Layer) with dimensions:, where K is the number of classes.Ĭonv2d_1 (Conv2D) (None, 126, 126, 32) 9248.Like conventional neural-networks, every node in this layer is connected to every node in the volume of features being fed-forward.'convolved features', are passed to a Fully-Connected Layer of nodes. A down-sampling strategy is applied to reduce the width and height of the output volume.The dimensions of the volume are left unchanged.
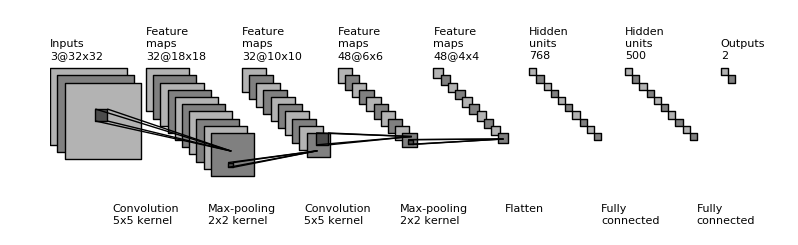

Layer is fed to an elementwise activation function, commonly a Rectified-Linear Unit (ReLu).
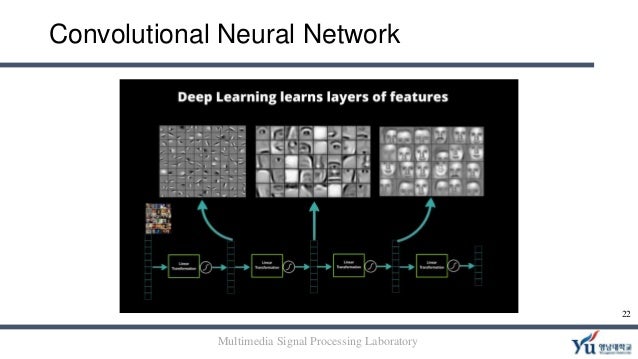
Recall that CNN architecture contains some essential building blocks such as:
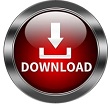